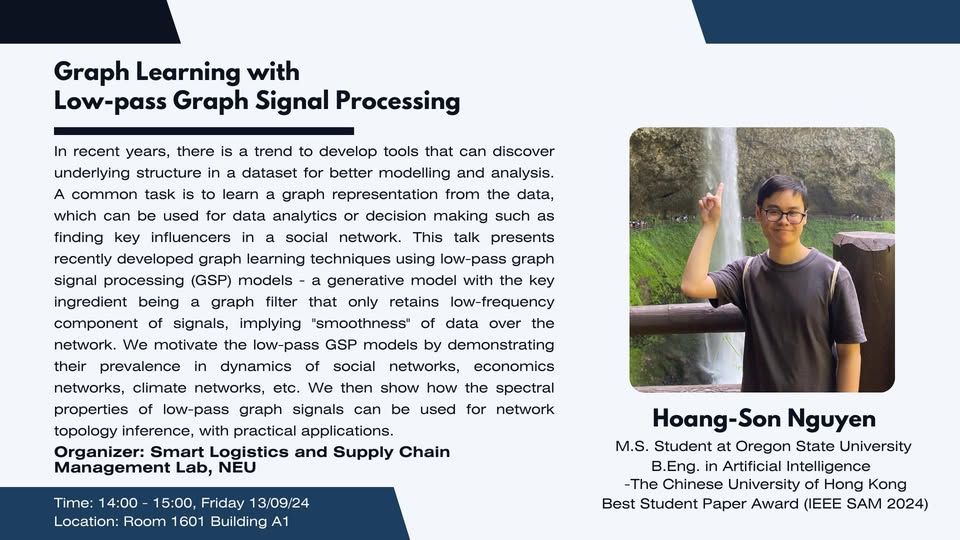
About The Event
Abstract: In recent years, there is a trend to develop tools that can discover underlying structure in a dataset for better modelling and analysis. A common task is to learn a graph representation from the data, which can be used for data analytics or decision making such as finding key influencers in a social network. This talk presents recently developed graph learning techniques using low-pass graph signal processing (GSP) models – a generative model with the key ingredient being a graph filter that only retains low-frequency component of signals, implying “smoothness” of data over the network. We motivate the low-pass GSP models by demonstrating their prevalence in dynamics of social networks, economics networks, climate networks, etc. We then show how the spectral properties of low-pass graph signals can be used for network topology inference, with practical applications.
Bio: Hoang-Son Nguyen is an incoming M.S. student at Oregon State University, working with Prof. Xiao Fu. He received his B.Eng. in Artificial Intelligence from The Chinese University of Hong Kong in March 2024, working with Prof. Hoi-To Wai on signal processing and machine learning over networks. He has received a Best Student Paper Award from IEEE SAM 2024. His current research focuses on principled methods for matrix factorization and unsupervised deep learning, with provable guarantees on identifiability and robustness.
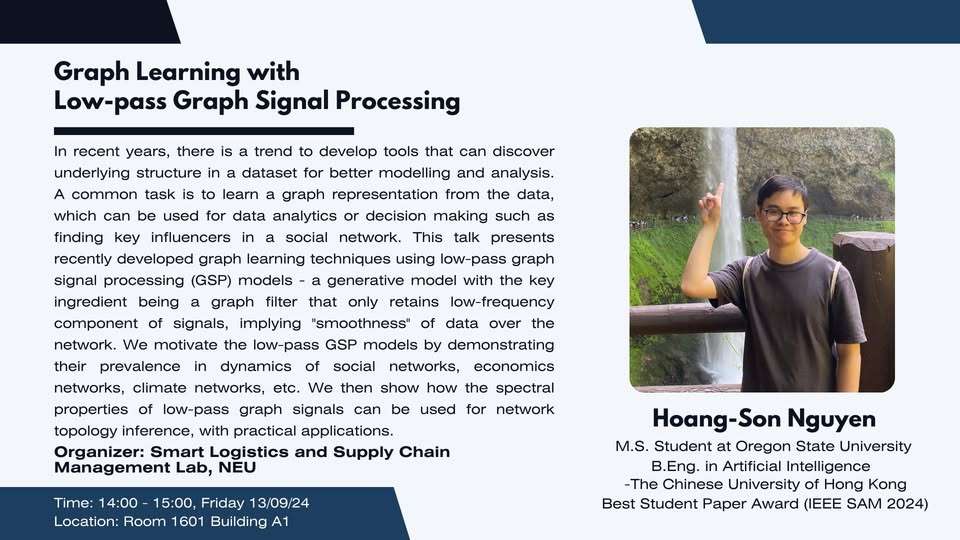